Bridging the Gap: How Model Metering and Security Can Drive On-Prem and VPC ML Revenue


Introduction
In the rapidly evolving landscape of machine learning (ML), on-premises and VPC deployments have become preferred choices for enterprises. The reasons? Enhanced data sovereignty, compliance, and security. However, this shift poses challenges, especially concerning model metering and Intellectual Property (IP) infringement detection.
Why On-Prem and VPC ML Deployments are Gaining Traction
On-prem and VPC ML deployments offer enterprises the autonomy they seek. By hosting ML models within their infrastructure or private cloud environments, they retain full control over data pipelines, ensuring data privacy and meeting stringent regulatory requirements. This approach mitigates the risks associated with data transit and external breaches.
Unlocking New Revenue Streams
In this dynamic landscape, solutions that address these challenges not only ensure security and compliance but also unlock new revenue streams. By facilitating secure on-prem deployments and offering robust metering capabilities, businesses can tap into previously inaccessible markets. This means more deployments, greater reach, and ultimately, more revenue.
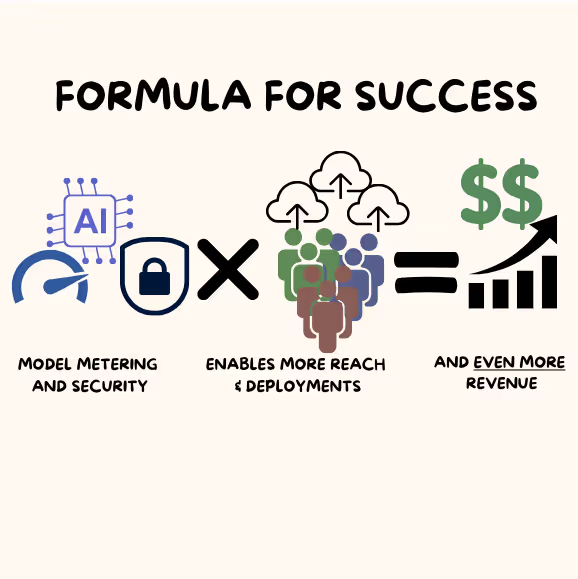
The Importance of Robust Metering for Revenue Assurance
For ML developers and providers, metering is paramount:
- Usage Analytics: Gaining insights into how frequently the model is accessed, and by which systems or users.
- Billing Accuracy: Ensuring that the model's consumption aligns with licensing agreements, preventing revenue leakage.
- Quota Management: Setting and tracking limits on model usage, based on enterprise agreements.
- Entitlements: Managing and enforcing the rights and privileges associated with model access, ensuring that only authorized users or systems can utilize the model.
The Roadblocks: Security and IP Infringement Detection Concerns
With metering in place, the next concern is security:
- IP Infringement Detection: The potential for proprietary models to be replicated or misused, and the capability to detect such infringements.
- Reverse Engineering: The threat of competitors or malicious actors deconstructing a model to understand its architecture and data.
The Role of Enhanced Model Security in On-Prem and VPC Deployments
To counter these challenges, advanced model security protocols are paramount:
- Encryption: Utilizing state-of-the-art encryption techniques to secure both model parameters and the data they process.
- Obfuscation: Making the model's internal workings ambiguous, deterring reverse engineering attempts.
- Runtime Protection: Ensuring the model operates securely and as intended during inference, preventing tampering or unauthorized access.
Spotlight: Enkrypt AI's Solutions for On-Prem and VPC ML Deployments
Enkrypt AI stands at the forefront of addressing these challenges. Enkrypt AI's suite of tools prioritizes:
- Comprehensive Metering Solutions: Providing real-time analytics on model usage, ensuring compliance, revenue assurance, and entitlement enforcement.
- IP Infringement Detection: Enkrypt AI focuses on detecting instances where models are being used in violation of licensing agreements, ensuring timely action.

Conclusion: The Future of On-Prem and VPC ML Deployments
The trajectory for on-prem and VPC ML deployments is clear: as the demand for data privacy and security grows, so will the need for advanced model metering and infringement detection solutions. With pioneers like Enkrypt AI leading the charge, the ML community is poised to navigate these challenges, unlocking new revenue streams in the process.