AI Hallucinations: Meaning, Causes, Real Life Examples & Best Ways to Prevent LLM Hallucinations in 2025
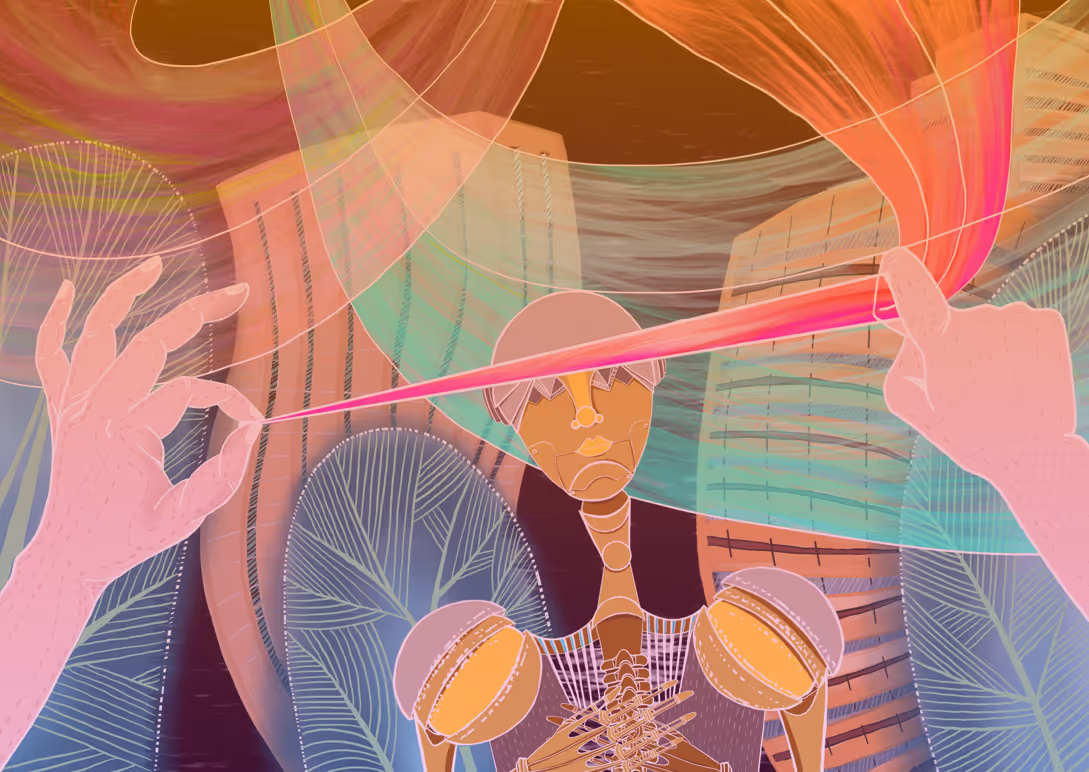
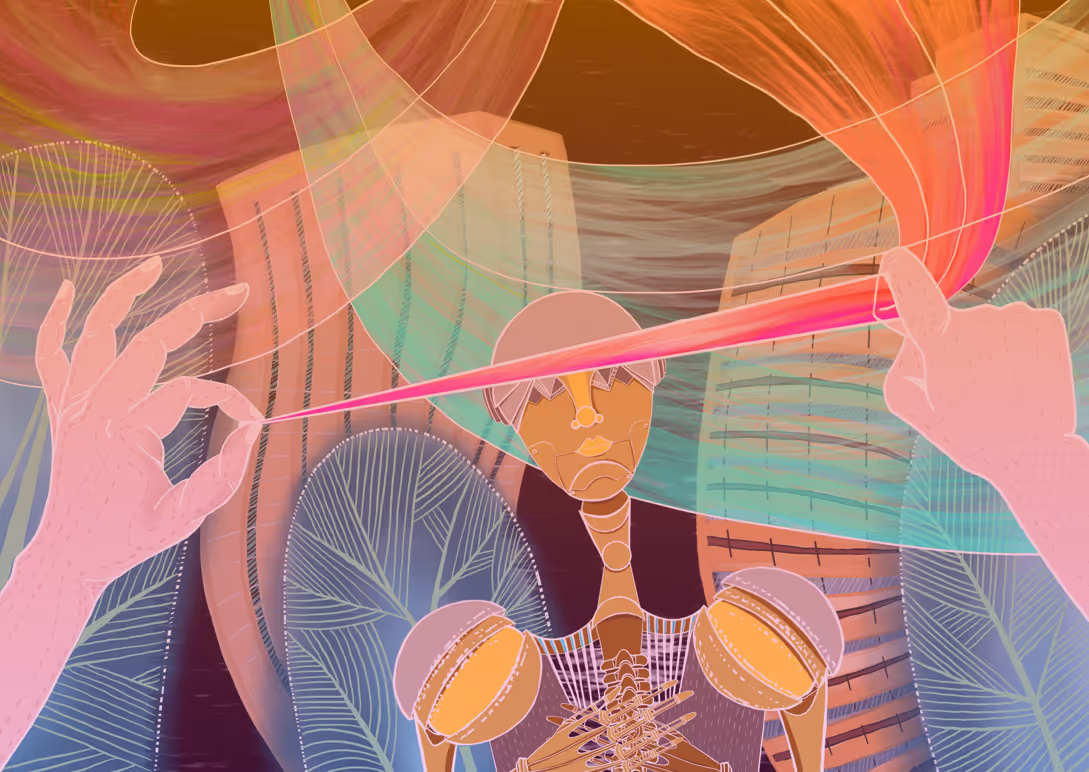
Introduction
As generative AI becomes increasingly integrated into high-stakes fields like healthcare, finance, and customer service, the issue of AI hallucinations (when an AI generates plausible but incorrect information) poses a significant risk. These inaccuracies have serious consequences, making it essential to address the root causes and develop effective mitigation strategies.
In this blog, we explore the causes of AI hallucinations and methods for preventing them.
What are AI Hallucinations?
AI hallucination is a phenomenon where an AI model generates outputs that are incorrect, nonsensical, or entirely fabricated, yet presents them as factual or accurate. This happens when the model perceives patterns or objects that are nonexistent or misinterprets the data it processes. The phenomenon arises due to factors like insufficient or biased training data, overfitting, or the model's inherent design, which prioritizes predicting plausible text rather than reasoning or verifying facts.
Example:
For instance, if asked about a fictional event, an AI might confidently assert, “The Moonlight Treaty was signed in 1854 between the U.S. and France,” even though no such treaty exists. This fabricated response, presented as factual, is an example of AI hallucination.
What Causes AI Hallucinations?
AI hallucinations often stem from limitations in the training data used for large language models. When a query requires current or specific knowledge not embedded in the model, the AI may generate responses based on plausible sounding but inaccurate information. In Retrieval Augmented Generation (RAG) applications, this problem is amplified by several factors:
- Context Misalignment: The model retrieves information that is irrelevant to the query, leading to confusion.
- Redundant or Conflicting Information: Retrieved passages may include extraneous data that distracts from, or contradicts, the correct answer.
- Incomplete or Outdated Content: If the retrieved information lacks completeness, the model may fill gaps based on previous patterns rather than on facts, resulting in inaccuracies.
Addressing these hallucinations is crucial for ensuring AI applications can reliably support users in high-stakes environments where precision is paramount.
Real-Life Examples of AI Hallucinations
Read some of the latest examples below, as many were taken from our AI Blunders site that you can peruse here.
· Legal Case Hallucinations: A New York lawyer cited six fake cases generated by ChatGPT in a legal brief, leading to potential sanctions. Despite assurances from ChatGPT about the validity of the cases, they were found to be completely fabricated, prompting a cautionary response from legal professionals.
· Air Canada’s Chatbot Error: Air Canada's AI tool gave incorrect advice for securing bereavement fare tickets, leading to legal action and partial refunds due to reputational damage.
· NYC Business Advice: The MyCity chatbot in New York City wrongly encouraged illegal practices, such as underpaying employees and stealing tips.
· Microsoft’s Tay Tweets: The Tay chatbot learned and posted inappropriate content on Twitter due to user manipulation, forcing Microsoft to shut it down within 24 hours.
· Amazon’s Biased Hiring Tool: An AI recruiting tool by Amazon discriminated against women due to biased training data, penalizing resumes containing words like "women’s."
· Google Image Misclassification: Google's AI misclassified images of Black people as gorillas, leading to removal of the feature.
· Driverless Car Accident: A GM Cruise car critically injured a pedestrian and mishandled the incident, dragging the victim further.
· War Crime Evidence Deletion: Social media AI deleted graphic content documenting war crimes, potentially obstructing justice for victims.
· Medical Chatbot Advice: A chatbot for eating disorder support offered harmful advice shortly after replacing human staff.
· Deepfake Financial Fraud: A British company lost over $25 million after an employee was duped by a voice deepfake into transferring funds.
· Character.AI Lawsuit: Parents sued Character.AI after its chatbot allegedly encouraged violent behavior and provided harmful content to children.
· Zillow’s AI Misstep: Zillow’s AI-powered home-buying system caused massive financial losses, leading to layoffs of 2,000 employees.
· Discriminatory Algorithms: The Dutch government’s AI falsely flagged over 20,000 families for welfare fraud, leading to mass resignations in parliament.
These cases underscore the need for rigorous verification, oversight, and ethical guidelines in AI deployment to prevent real-world consequences.
What is the Impact of Hallucination in LLMs?
AI hallucinations, while not yet fully preventable, underscore the need for caution, rigorous verification, and ethical design in deploying AI across various functions.
Misinformation and Trust Erosion
AI hallucinations can disseminate erroneous or misleading information, undermining user trust. In important situations, such as crises or healthcare, they can aggravate misinformation, resulting in poor decision-making or dangerous behavior.
Bias Amplification
Models trained on biased datasets may hallucinate patterns that reinforce existing biases, exacerbating discrimination in areas such as hiring, lending, and law enforcement.
Adversarial vulnerabilities
Adversarial attacks can influence AI outputs, raising hazards in fields such as cybersecurity and self-driving cars. Subtle input changes can result in significant misclassifications.
Brand and Reputational Risks
a. AI hallucinations in customer-facing interactions can damage brand identity by spreading inaccurate or inconsistent information.
b. Misleading promises, such as refunds or bonuses, can lead to either financial losses or reduced customer loyalty if unfulfilled.
Costly Business Errors
Companies may face financial and legal liabilities as a result of hallucinated outputs, as demonstrated by the Air Canada case, when hallucinated policies resulted in reputational damage and legal fees.
Ill-informed Decision-Making
Decisions based on hallucinated data can result in negative outcomes:
· Low-risk scenarios: Errors in marketing initiatives may have little impact.
· High-risk scenarios: Decisions like closing financial accounts or automating critical tasks can cause significant financial and reputational damage.
Operational and Ethical Concerns
AI hallucinations challenge operational reliability across business functions, from marketing to HR and finance. They highlight the importance of robust training, fact-checking, and human oversight in AI applications.
10 Best Ways to Prevent LLM Hallucinations in 2025
AI hallucinations, the phenomenon where AI systems generate incorrect or nonsensical outputs, are challenging to eliminate entirely. However, with advancements in AI development and strategic approaches, we can significantly reduce their occurrence. Below are some effective ways to minimize AI hallucinations.
Utilize Retrieval-Augmented Generation (RAG)
RAG integrates AI models with reliable databases, enabling them to access accurate information in real-time. This approach is commonly used in tools designed for specialized tasks, such as citing legal precedents or responding to customer queries using verified sources. While RAG is not foolproof, it’s a widely adopted method for enhancing AI accuracy.
Leverage High-Quality Training Data
The quality of an AI model's training data is critical. Ensure the data is diverse, well-structured, and relevant to the model’s purpose. This helps the AI avoid biases, better understand tasks, and produce accurate outputs.
Define Clear AI Model Objectives
Establishing well-defined purposes and limitations for your AI system minimizes irrelevant outputs. When the AI’s tasks and boundaries are clear, the likelihood of LLM hallucination decreases significantly.
Incorporate Prompt Engineering
How you interact with AI can influence its accuracy.
· Be Specific: Provide detailed instructions and relevant context to guide the AI effectively.
· Use Chain-of-Thought Prompts: Break down complex tasks into smaller steps and ask the AI to explain its reasoning.
· Limit Outcomes: Frame prompts with constrained options or examples to reduce the scope for hallucinations.
Implement Data Templates
Predefined data templates help guide AI outputs, ensuring they align with expected formats. For instance, in text generation tasks, templates can enforce a consistent structure, reducing errors.
Regular Testing and Refinement
Continuous evaluation and refinement are essential for maintaining AI accuracy. Regularly test the system with updated data to identify and address potential weaknesses to prevent AI hallucinations in 2025.
Set Output Constraints
Limit the range of potential outputs using probabilistic thresholds or filtering tools. This approach helps keep AI responses consistent and aligned with the task requirements.
Employ Human Oversight
Human validation acts as the ultimate safeguard against AI hallucinations. Experts can review AI outputs for relevance and accuracy, correcting errors and enhancing the overall quality of results.
Fact-Check and Verify Outputs
Even with advanced tools, verification is crucial to prevent LLM hallucinations. Always cross-check AI-generated outputs, especially for critical tasks, to ensure accuracy and reliability.
Train AI on Specific and Relevant Sources
Using specialized, domain-specific datasets tailored to the AI’s purpose minimizes the risk of hallucination. For example, medical AI should rely on verified medical data for training.
Establish Feedback Loops
Encourage iterative feedback to improve AI performance. By informing the system about accurate and inaccurate outputs, you can enhance its learning over time.
How Can Enkrypt AI Help Prevent AI Hallucinations?
Our team at Enkrypt AI developed a novel approach for preventing AI hallucinations through a new step-by-step validation process that detects and removes hallucinations in two specific ways:
- Pre-Response Validation: The platform assesses whether retrieval is necessary for the given query. It proceeds with retrieval only when external information is needed and evaluates the retrieved context to eliminate any irrelevant, redundant, or conflicting information that could mislead the model.
- Post-Response Refinement: After generating a response, the platform decomposes it into atomic statements and analyzes each for accuracy against the retrieved data. Any statements that stray from the context or contain superfluous details are edited or removed, resulting in concise, contextually grounded answers.
See Figure 1 below, illustrating this 2-step approach to AI hallucination prevention.
.avif)
You can also see an example of how AI hallucinations can be prevented in our product demo video below.
Video: Preventing AI Hallucinations
Effectiveness of Our AI Hallucination Prevention Capability in RAGs
We measured the effectiveness of our AI hallucination prevention capability in RAGs using three key metrics:
- Response Adherence: This measures how closely the model’s response aligns with the provided RAG context.
- Response Relevance: This checks the degree to which the information in the model's response directly answers the query, minimizing extraneous details.
- Context Relevance: This evaluates the relevance of the retrieved context to the query.
An increase in these metrics directly correlates to a reduction in AI hallucinations, demonstrating our platform’s effectiveness on various RAGs, as shown in Figure 2 below.
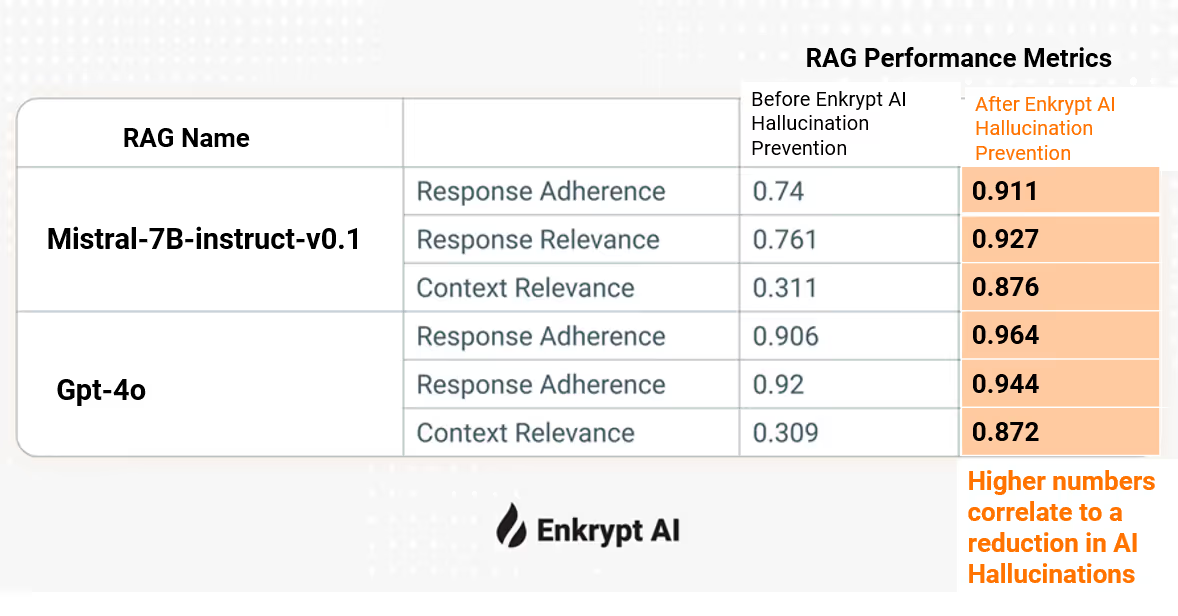
Conclusion
Enkrypt AI’s hallucination prevention capability employs a multi-layered approach to effectively detect and remove AI hallucinations by refining both context and responses. By implementing our solution, users can expect improved accuracy and reliability in AI applications.
We are excited to announce that a detailed technical paper will be published soon, offering deeper insights into our findings.
For those interested in building reliable generative AI applications, we invite you to learn more and request a demo at Enkrypt AI.
FAQs
1. What are LLM Hallucinations?
Answer: LLM hallucinations are errors that arise when a large language model (LLM) produces replies that are inaccurate, irrelevant, or illogical. They arise from the probabilistic nature of these models, which rely on patterns in training data rather than true insight.
2. What are some real-world examples of AI hallucinations that cause harm?
Answer: Here are some real-world examples of AI hallucinations that caused harm below:
· Microsoft Travel Article Error: AI listed Ottawa Food Bank as a "tourist hotspot," causing public embarrassment and trust issues.
· Google Bard's Public Demo Mistake: Incorrectly credited James Webb Space Telescope with a discovery, leading to a $100 billion stock drop.
· Bing Chat Financial Inaccuracies: Gave erroneous financial data, weakening trust.
· Legal Precedent Fabrication: Lawyer cited non-existent cases created by ChatGPT, resulting in a $5,000 fine.
· False Israel-Hamas Ceasefire: Bard and Bing Chat falsely claimed a ceasefire, showcasing misinformation risks.
· Amazon's Mushroom Foraging Guides: AI-generated books contained dangerous misinformation about identifying edible mushrooms.
3. How does Enkrypt AI prevent AI hallucinations in RAG applications?
Answer: Enkrypt AI employs a two-step approach to prevent AI hallucinations:
· Pre-Response Validation: Ensures that retrieval is necessary for a query and eliminates irrelevant, redundant, or conflicting information before generating a response.
· Post-Response Refinement: Decomposes the generated response into atomic statements, analyzing each for accuracy against the retrieved data to ensure concise and contextually grounded answers.
4. Is it possible to totally prevent AI hallucinations?
Answer: While it is impossible to completely avoid AI hallucinations, they can be reduced with the use of strategies like reinforcement learning with user feedback, high-quality training data, and retrieval-augmented generation (RAG).
5. What is the best strategy to reduce hallucinations caused by AI?
Answer: One of the best approaches to reduce hallucinations is to use RAG, which gives the AI access to reliable databases. It guarantees that answers are supported by actual data.