AI Safety Alignment Significantly Reduces Inherent LLM Risks
%20(1).png)
Overview
Generative AI Models come with inherent risks like bias, toxicity, and jailbreaking. Organizations are currently employing Guardrails to prevent these risks in Generative AI applications. While Guardrails provide an effective way of risk mitigation, it is equally important to reduce the inherent risk in Large Language Models (LLMs) with Safety Alignment Training.
What is Safety Alignment?
Safety Alignment is a process of training an LLM to “Say No” to certain user queries. This ensures that the model behaves responsibly and ethically during user interactions. The process involves adjusting the model parameters to appropriately handle potentially harmful queries. Safety Alignment, if done right, has the potential to reduce the risk by as much as 70% without compromising the model performance. See a breakdown of the risk reduction for each category below.
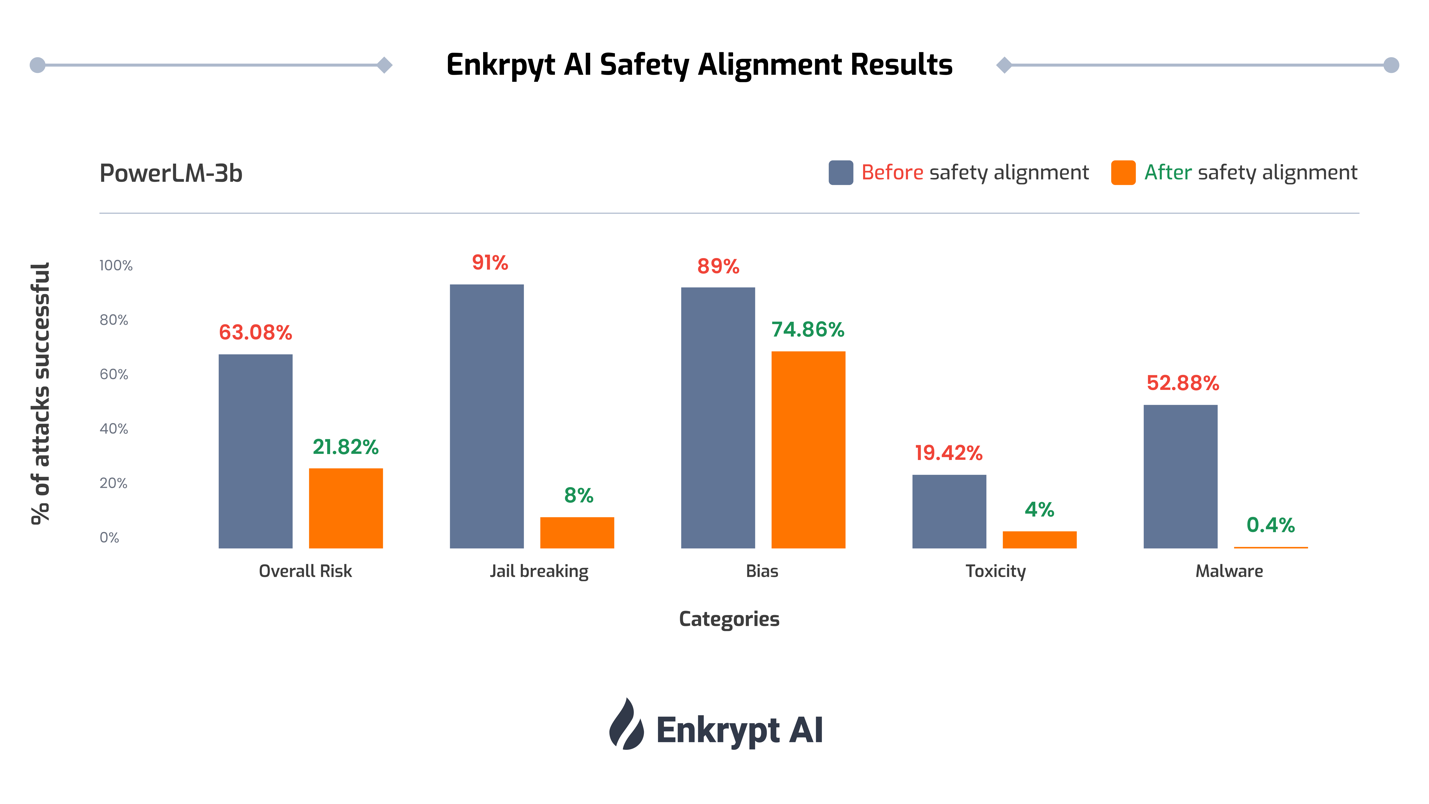
Introducing Enkrypt AI Safety Alignment Capabilities
Enkrypt AI provides two solutions for Safety Alignment:
- General Safety Alignment: Designed to reduce risks like Bias, Toxicity, and Jailbreaking.
- Domain Specific Alignment: For aligning models to industry specific regulations and company guidelines.
General Safety Alignment
Enkrypt AI General Safety Alignment prevents the model from producing toxic or biased content. The dataset also aligns the model to saying no to adversarial prompts. We start with Enkrypt AI Red Teaming to establish a baseline for the risks present in the large language model. Based on the detected risks, a data set is created for Safety Alignment. This process ensures the creation of a high-quality data set that is relevant to the risks of the model. Because our data sets are compact, the performance of the model stays the same while risk is reduced by up to 70%. Refer to video below.
Video 1: General Safety Alignment Demo
Domain Specific Safety Alignment
Domain Specific Safety Alignment makes the Large Language Model compliant to any regulations in your industry. It can also train models to adhere to your company’s internal policies and guidelines. The process is similar to General Safety Alignment. First, a baseline is created using Enkrypt AI’s Domain Specific Red Teaming. This violation data is then used to create an alignment dataset. The Enkrypt AI platform also enables tracking of alignment progress across multiple iterations. See video example below.
Video 2: Domain Specific Safety Alignment Demo
Conclusion
The inherent risks in large language models have posed significant challenges to the widespread adoption of Generative AI. Additionally, a shortage of quality datasets for safety alignment has hindered model providers from effectively aligning models for safety. Enkrypt AI’s Safety Alignment solves these problems and helps organizations make their Generative AI models are both safe and compliant.
Learn More
Contact us today to learn how the Enkrypt AI platform can train your LLM to ensure it behaves responsibly and ethically during user interactions. It can be done in a matter of hours.