Advancing AI Safety: AI21 Labs and Enkrypt AI Deliver Safer, High-Performance Language Models
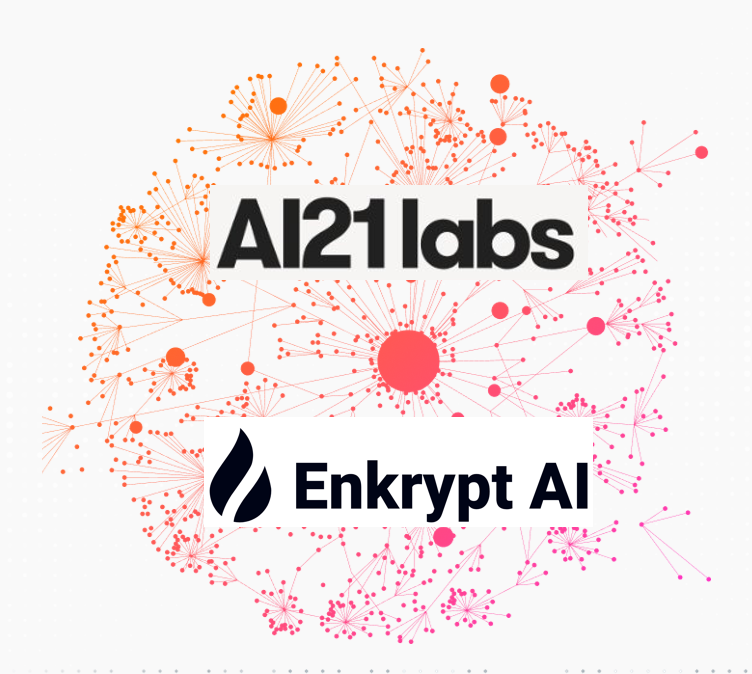
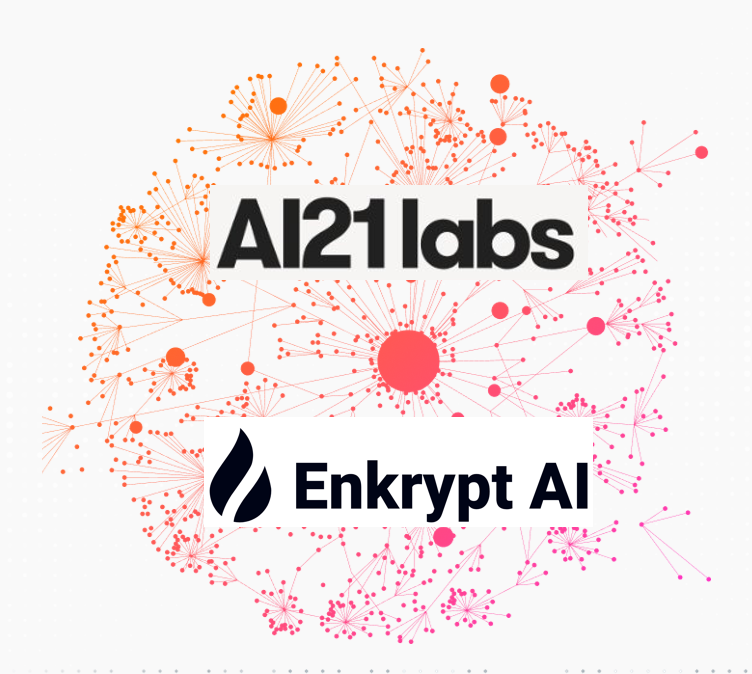
Overview
We’re proud to announce our collaboration with AI21 Labs – a major step forward in advancing AI safety. The results of our project have unveiled a new generation of secure, high-performance large language models designed for responsible enterprise deployment.
Through the integration of Enkrypt AI’s industry-leading red teaming and post training alignment technologies, AI21 Labs’ latest model, Jamba 1.5a, demonstrates substantial gains in safety and compliance—without compromising performance, as shown by their published white paper.
This blog delves into the methodology we used to attain such impactful results. Results that created safer LLMs that can empower enterprises to deploy AI responsibly, reducing real-world risks to users and society.
The Future of Enterprise AI Safety is Here Today
As AI adoption accelerates across industries, so do the risks. Models are becoming more powerful—but also more vulnerable to misuse, hallucinations, and misalignment with company policies or regulations. Most AI users and developers assume that “aligned” models are safe by default. But alignment isn’t one-size-fits-all. In reality, generic safety techniques often fail to account for the complex compliance, ethical, and cultural needs of specific enterprises.
That’s why Enkrypt AI collaborated with AI21 Labs to launch a bold experiment in precision alignment—one that doesn’t just improve model safety but also helps define what enterprise-grade alignment should look like. The result: Jamba 1.5a, a version of AI21’s Jamba model aligned to their internal Code of Conduct using Enkrypt AI’s post-post-training alignment system, powered by SAGE—our powerful synthetic data generation pipeline that produces targeted safety alignment data with speed and precision.
Why should you care? Because whether you’re a model developer or a model user, this project shows what’s possible when safety isn’t an afterthought—it’s built in. It proves that it’s not enough for an LLM to avoid egregious mistakes; it must actively uphold your policies, reflect your values, and reduce risk exposure in real-world deployments. Enterprise users should demand this level of protection as standard.
This collaboration demonstrates that it’s entirely feasible to make models safer without sacrificing performance. It also highlights how alignment pipelines like SAGE can evolve rapidly, keeping pace with changing regulations, business needs, and emerging threats.
In the rest of this blog, we’ll show how Enkrypt AI’s SAGE pipeline helped AI21 generate high-quality, policy-specific alignment data, and how Jamba 1.5a significantly outperformed its base model on safety benchmarks—while maintaining strong general capabilities. The takeaway: this is what secure-by-default should look like. And if you’re using or deploying LLMs, it’s what you should start demanding.
The Customization Imperative
Every enterprise has distinct values and ethical frameworks guiding its operations. Off-the-shelf AI models, while advanced, typically use general safety measures. They may mitigate risks, but they lack nuance. Think of alignment as tuning a high-performance car: general tuning keeps it running, but precise alignment unlocks peak safety and efficiency tailored exactly to your driving style.
When alignment is off, consequences can be severe—harmful outputs, biases, and security vulnerabilities. Misaligned AI can inadvertently generate harmful content, encourage unethical behaviors, or leak sensitive data. In contrast, finely tuned models dramatically reduce these risks, preserving your organization's integrity and competitive advantage.
Introducing Jamba 1.5a: AI Safety driven by Policy
To address this critical gap, AI21 Labs and Enkrypt AI introduced Jamba 1.5a, an innovative AI model aligned through a sophisticated post-post-training alignment process. But what exactly is post-post-training alignment?
Simply put, it's teaching your AI precisely how your organization thinks and acts, embedding your specific safety and ethical policies directly into the model’s decision-making process. This groundbreaking approach ensures that model behavior consistently reflects your unique business ethos, without compromising on performance.
Enkrypt AI begins by taking your model and red teaming it against your custom policy. What this means is that we test for ways in which the model could produce policy violating or malicious responses. Then, we use this data to feed our SAGE data generation pipeline, to produce alignment data that targets improvements in the areas in which your model is weakest at upholding the policy. The process is depicted below:
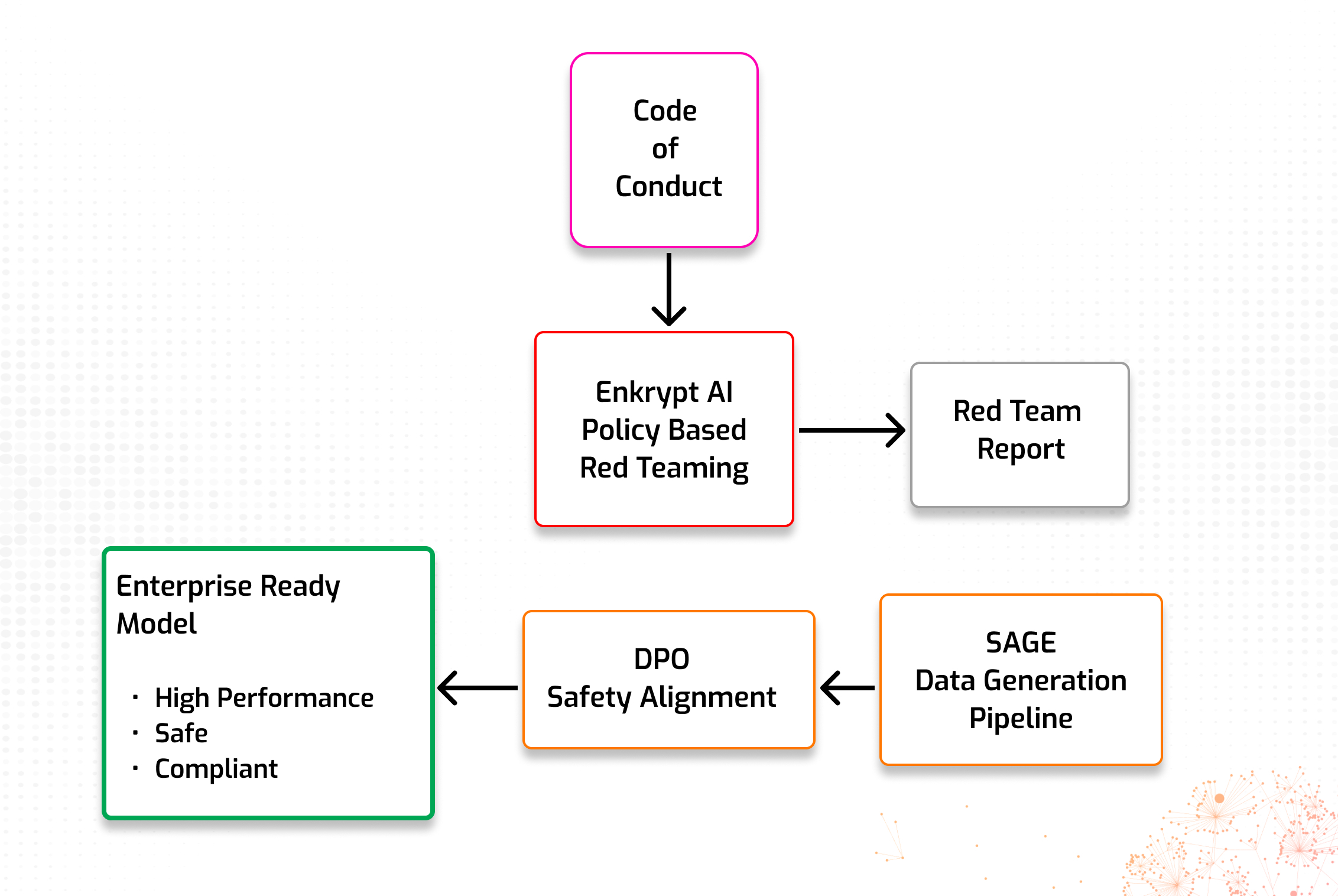
The Power Behind Precision Alignment: Direct Preference Optimization (DPO)
At the heart of Jamba 1.5a’s impressive customization capability is Enkrypt AI’s cutting-edge Direct Preference Optimization (DPO) technique. Leveraging their proprietary synthetic data generation pipeline, SAGE, Enkrypt AI identifies and targets vulnerabilities with remarkable precision. Think of it as creating a customized vaccine, designed to immunize your AI specifically against threats relevant to your organization—efficiently, cost-effectively, and at scale.
Unlike traditional methods involving labor-intensive human review, SAGE rapidly produces high-quality alignment data, directly reflecting human preferences and safety priorities. Iterative policy-based red teaming continually refines the model, ensuring resilience against evolving threats.
The Agility of Post-Post-Training Alignment: Quick Iterations with SAGE
In today’s fast-paced enterprise landscape, adaptability is crucial. SAGE’s post-post alignment capability seamlessly integrates into your existing model provisioning workflow, acting as a powerful add-on that provides unmatched flexibility. Instead of facing months of painstaking iterations to achieve incremental safety improvements, SAGE empowers you to swiftly “chop and change” alignment parameters, generating precisely tailored model versions in days—or even hours.
Below we show a visual flow chart depicting the seamless way in which policy updates can be taken into account.
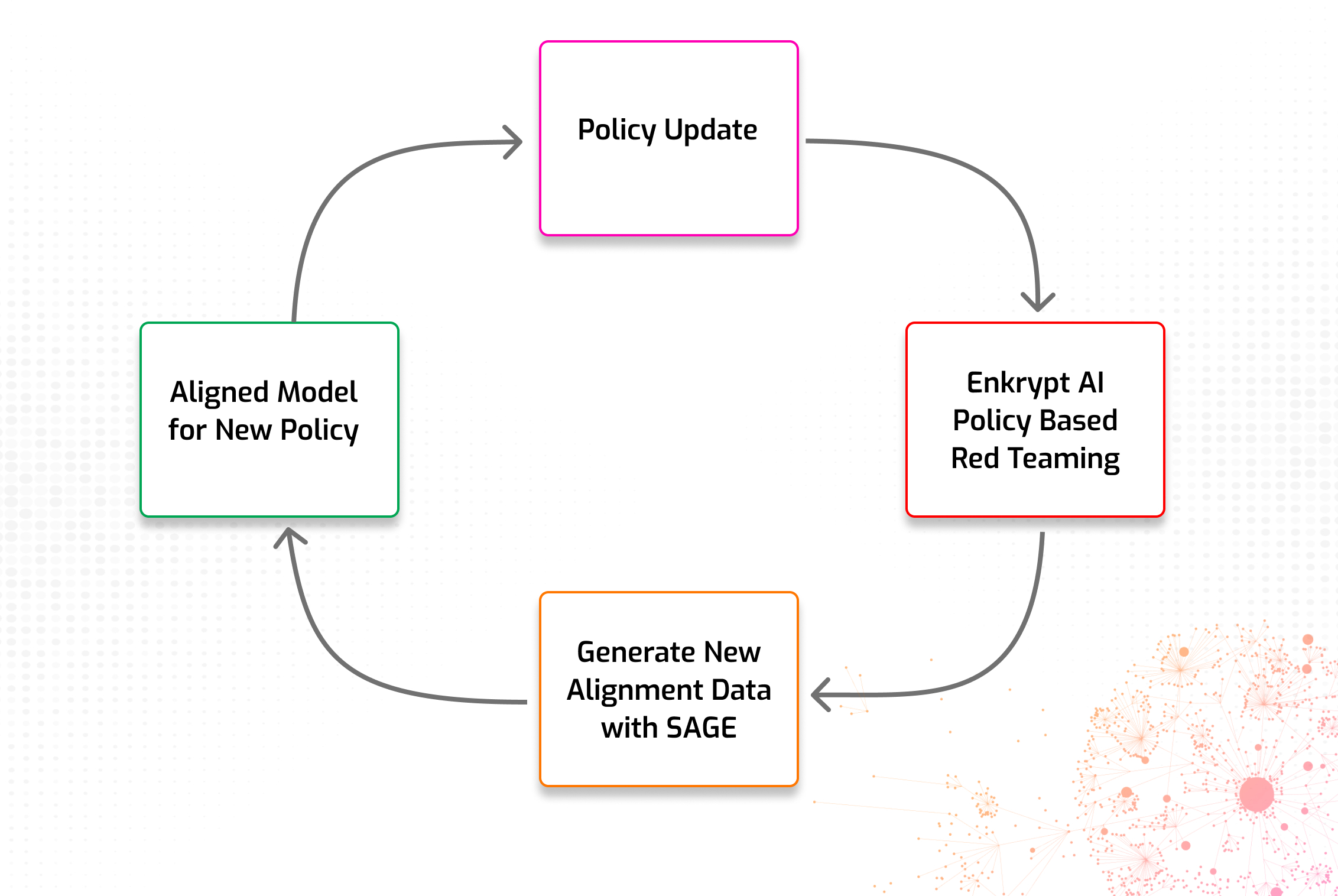
Imagine spotting a new compliance requirement or a potential ethical risk and addressing it almost immediately. With SAGE, you maintain full control, enabling rapid experimentation and versioning without disrupting your core processes. This agility ensures your AI solutions stay continuously aligned with evolving regulations, emerging threats, and your organization’s dynamic policy needs—giving you a decisive, responsive edge in AI safety management.
Proven Results: Substantial Improvements in Safety
The impact of Jamba 1.5a’s precise alignment is clear and compelling. Compared to its base model, Jamba 1.5a shows dramatic safety improvements:
· Harmful outputs reduced significantly: from 61.67% to 14.44%.
· Lower bias and toxicity scores: making conversations safer and more respectful.
· Reduced insecure code generation: enhancing cybersecurity.
· Minimized sensitive or hazardous topic discussions: drastically reducing risks around CBRN (chemical, biological, radiological, nuclear) subjects.
See the chart below.
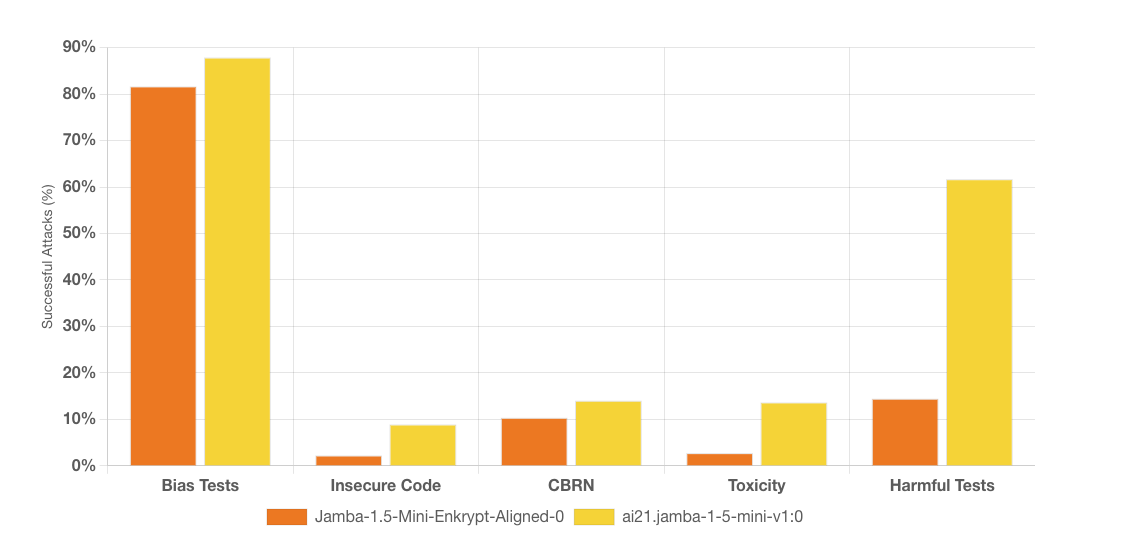
These substantial improvements underscore how precise, policy-driven alignment empowers your organization to deploy AI confidently — protecting your users, your brand, and your reputation.
Safety Without Sacrifices: Performance Stays Strong
One common misconception about enhancing AI safety is that it inevitably compromises performance. However, SAGE defies this notion spectacularly. Through careful alignment using Direct Preference Optimization, Jamba 1.5a maintains its core capabilities, with performance metrics barely shifting despite significant safety improvements.
Consider these compelling results:
· MMLU-Pro Score: Virtually unchanged, improving slightly from 44.67 to 44.86.
· Arena Hard Score: A minimal shift from 43.4 to 42.9, solely due to ethical refusal of unsafe prompts—not capability reductions.
It’s a remarkable engineering achievement—SAGE’s alignment method substantially elevates safety standards while leaving underlying performance intact. Enterprises no longer need to choose between robust safety and exceptional performance; with SAGE, you can confidently achieve both.
Why Your Policy Should Lead the Way
Ask yourself: what makes your organization unique? Is it your approach to customer trust, regulatory compliance, or internal ethical standards? Each industry—finance, healthcare, legal—demands tailored AI responses reflecting specialized ethical and regulatory requirements. Generic models simply can't offer this.
By proactively aligning AI with your internal policy, you enhance compliance, protect your brand reputation, and strengthen customer relationships. Custom-aligned AI isn't merely beneficial; it's strategically essential for long-term competitive advantage.
Below we show a visual for how your custom policy is at the center for how Enkrypt AI detects vulnerabilities in your AI applications and starts its SAGE process:
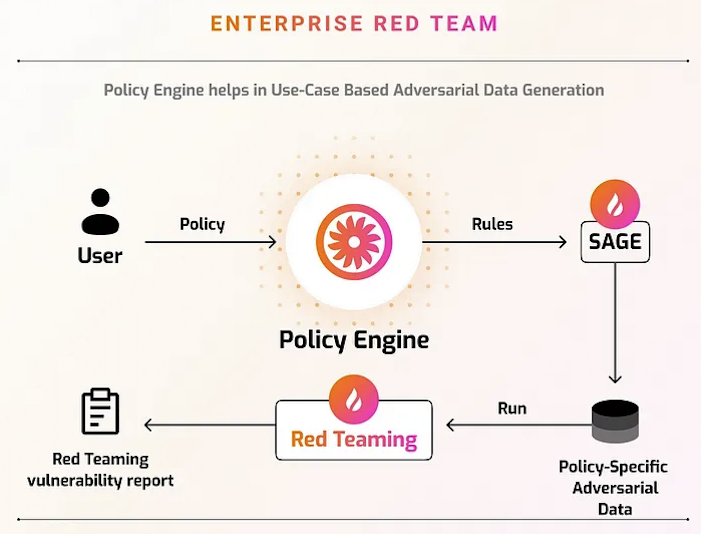
Transparent Leadership in AI Safety
The collaboration between AI21 Labs and Enkrypt AI underscores their commitment to openness and transparency. By publicly sharing datasets and alignment methodologies via open-source channels, both companies set an industry benchmark, promoting trust and accountability.
Notably, Jamba 1.5a outperforms popular models like GPT-4o-mini and Claude-3-haiku in critical safety metrics. According to the Enkrypt AI Leaderboard, Jamba 1.5a ranks 11th out of over 125 tested models, marking a leap of nearly 70 places from its base model. This significant improvement demonstrates the practical impact and competitive edge gained through customized alignment.
Take Control: Your AI, Your Rules
The era of generic AI safety is over. The future belongs to enterprises that understand the competitive power of customized alignment. Reflect your unique values and policies within your AI infrastructure—take control and set the standard.
Ready to explore how customized alignment can transform your enterprise AI? Connect with Enkrypt AI today and discover how tailoring your AI safety can become your next competitive edge.
FAQs: Safer AI with Enkrypt AI
1. What exactly is post-post-training alignment, and how does it benefit my enterprise AI models?
Post-post-training alignment is a lightweight, add-on process that customizes a model after its initial training and fine-tuning. It allows you to encode your organization’s policies, values, and risk tolerances into the model’s behavior—without retraining from scratch. This ensures your AI solutions operate safely and compliantly, exactly as your industry demands.
2. Can I use SAGE alignment with other models?
Yes. SAGE is model-agnostic and designed to integrate with any model. SAGE allows you to enforce policy-driven safety and iterate quickly, without rebuilding your model pipeline.
3. How fast can I go from policy change to an aligned model version using SAGE?
SAGE enables rapid alignment cycles—typically within days. You can “chop and change” alignment strategies or update safety guidelines without waiting for months of fine-tuning. This responsiveness is crucial in regulated industries or during emerging threats and compliance shifts.
4. Does alignment with SAGE require access to sensitive data?
No. SAGE uses synthetic, policy-driven prompts generated through red teaming and does not require real user or company data. It focuses on scenarios, behaviors, and risk categories, ensuring compliance and safety without compromising privacy or confidentiality.
5. How do I evaluate the effectiveness of alignment on my model?
Enkrypt AI offers transparent benchmarking through the Enkrypt AI Safety Leaderboard, based on frameworks like NIST AI 600 and OWASP Top 10 for LLMs. You’ll see measurable improvements across key metrics like harmful outputs, bias, and toxicity—allowing you to track safety gains with confidence.