Ensuring AI Safety and Compliance: Comparative Study of LLM Guardrails

As organizations increasingly incorporate large language models (LLMs) into their operations, it becomes crucial to implement robust guardrails to ensure the safe, reliable, and ethical operation of these powerful AI systems. Generative AI guardrails act as security and control mechanisms, mitigating various risks associated with the misuse or unintentional flaws of AI models. See figure 1 below.
.jpeg)
These risks span across several key areas: security, privacy, integrity, moderation, and compliance.
Understanding Key Risk Areas in LLM Deployment
To deploy LLMs responsibly, organizations must address risks across several domains:
- Security Risks
- Threats: Vulnerabilities like prompt injection—where malicious inputs manipulate an LLM’s outputs—can lead to harmful content generation or security breaches.
- Mitigation: Guardrails must detect and neutralize such threats in real-time.
- Privacy Risks
- Threats: AI models may inadvertently expose personally identifiable information (PII) or sensitive data through outputs.
- Mitigation: Guardrails ensure compliance with privacy standards by identifying and blocking potentially sensitive content.
- Integrity Risks
- Threats: LLMs can produce hallucinations—inaccurate or nonsensical outputs—that undermine trust and reliability.
- Mitigation: Features like hallucination detectors validate the accuracy and relevance of AI outputs.
- Moderation Risks
- Threats: The generation of offensive, biased, or inappropriate content can harm user trust and brand reputation.
- Mitigation: Robust moderation tools monitor for and filter inappropriate language.
- Compliance Risks
- Threats: Non-compliance with data regulations, ethical guidelines, or industry standards can result in fines and reputational damage.
- Mitigation: Guardrails enforce adherence to both internal policies and external regulations.
This study provides a detailed comparative analysis of guardrail providers for prompt injections—Enkrypt AI, IBM Granite, Azure AI Prompt Shield, and AWS Bedrock—focusing on their performance, features, and suitability for different organizational needs. Choosing the right guardrail provider is critical for mitigating risks, ensuring compliance, and safeguarding AI systems against misuse.
We first compare the performance of these guardrail providers for security threats, as shown in Figure 2 below. Each provider is evaluated using these criteria along with key performance metrics such as accuracy, precision, recall, F1 score, and average latency to offer a comprehensive view.
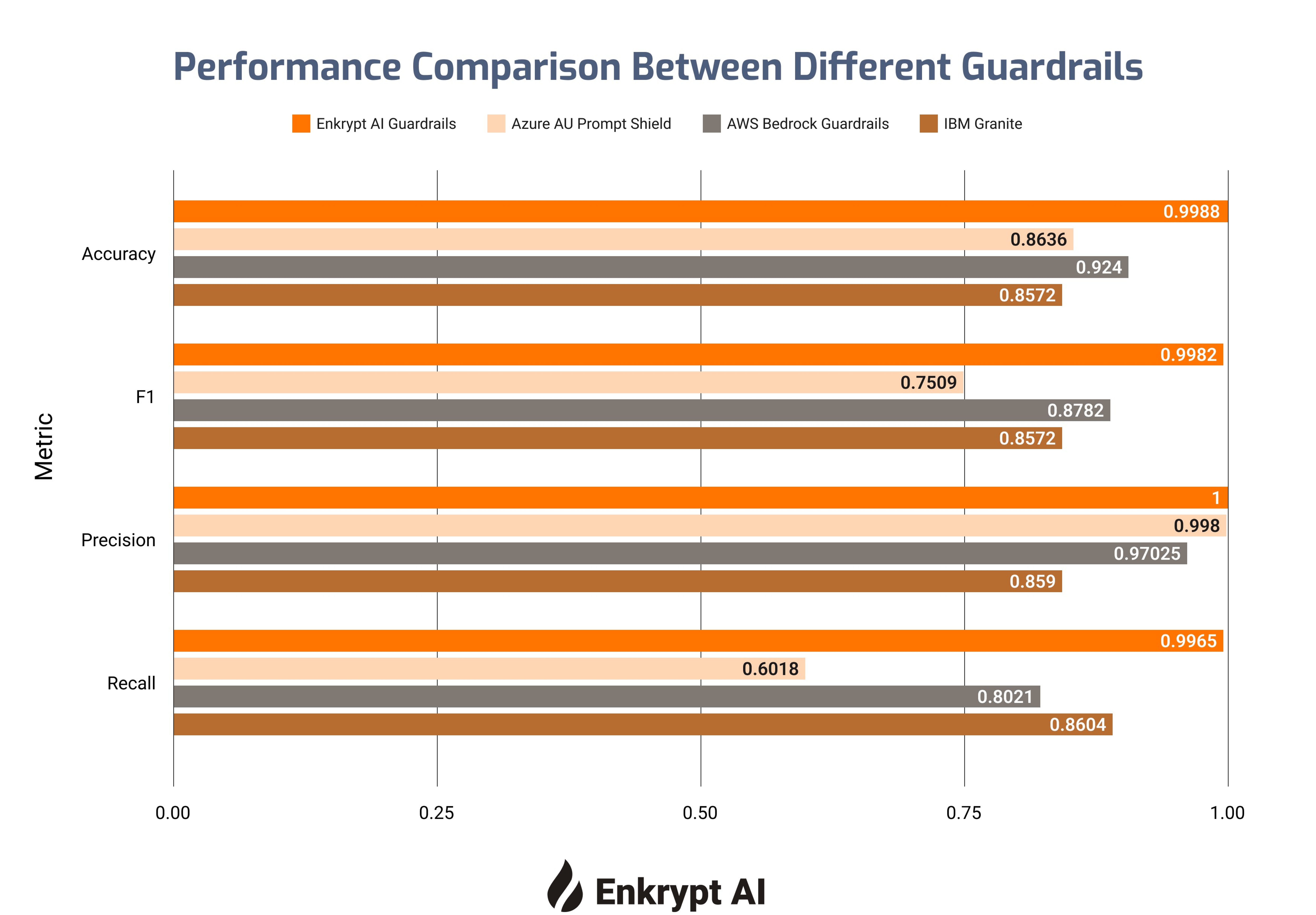
Guardrails are tested using prompts of varying token sizes to simulate real- world conditions. Tokens represent words or word fragments that LLMs process to generate outputs. Testing across different token sizes ensures scalability and reliability:
- Small Prompts (100-250 tokens): Reflect short-form, user-facing queries, as shown in Figure 3.
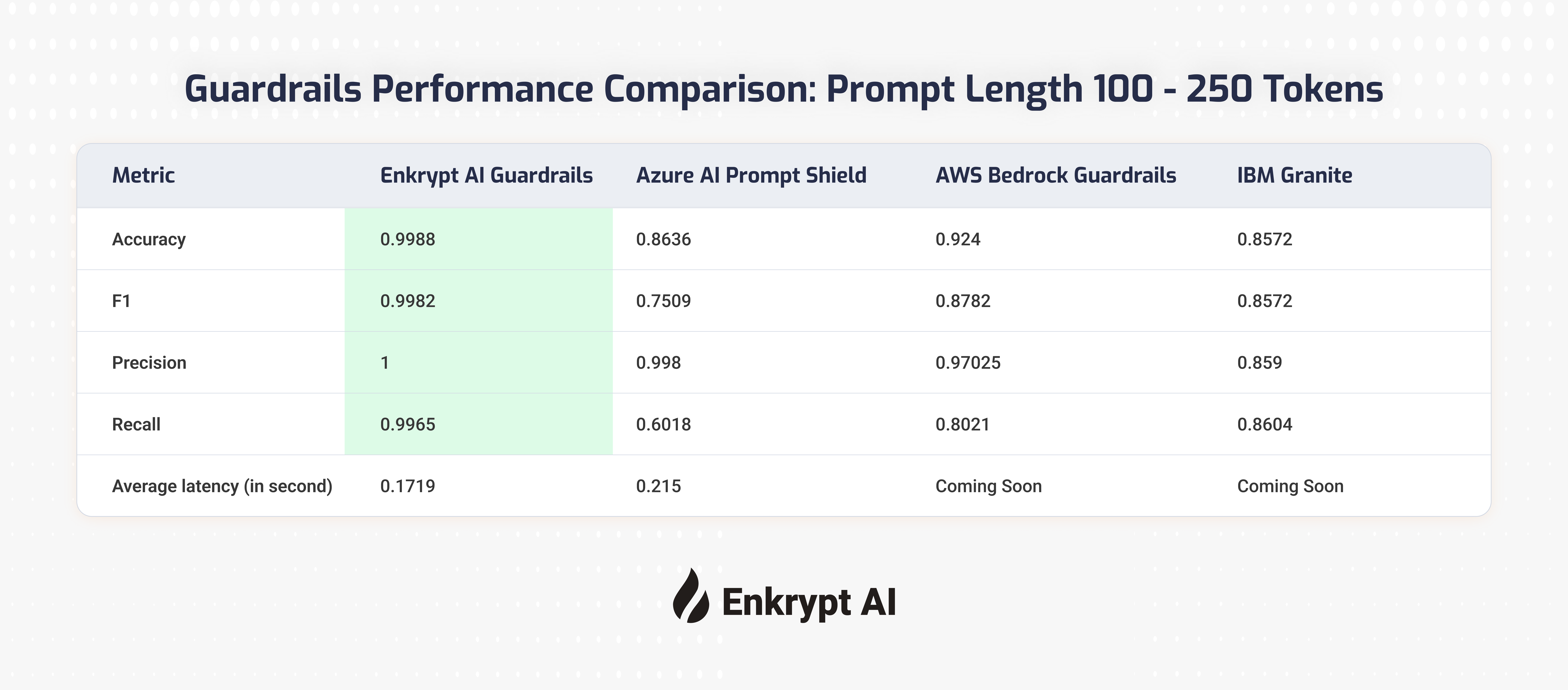
- Moderate Prompts (~632 tokens): Test performance with average- length requests, as shown in Figure 4.
.png)
- Large Prompts (up to 7,000 tokens): Assess stability and performance under high-load conditions, such as document analysis or bulk data processing, as shown in Figure 5 below.
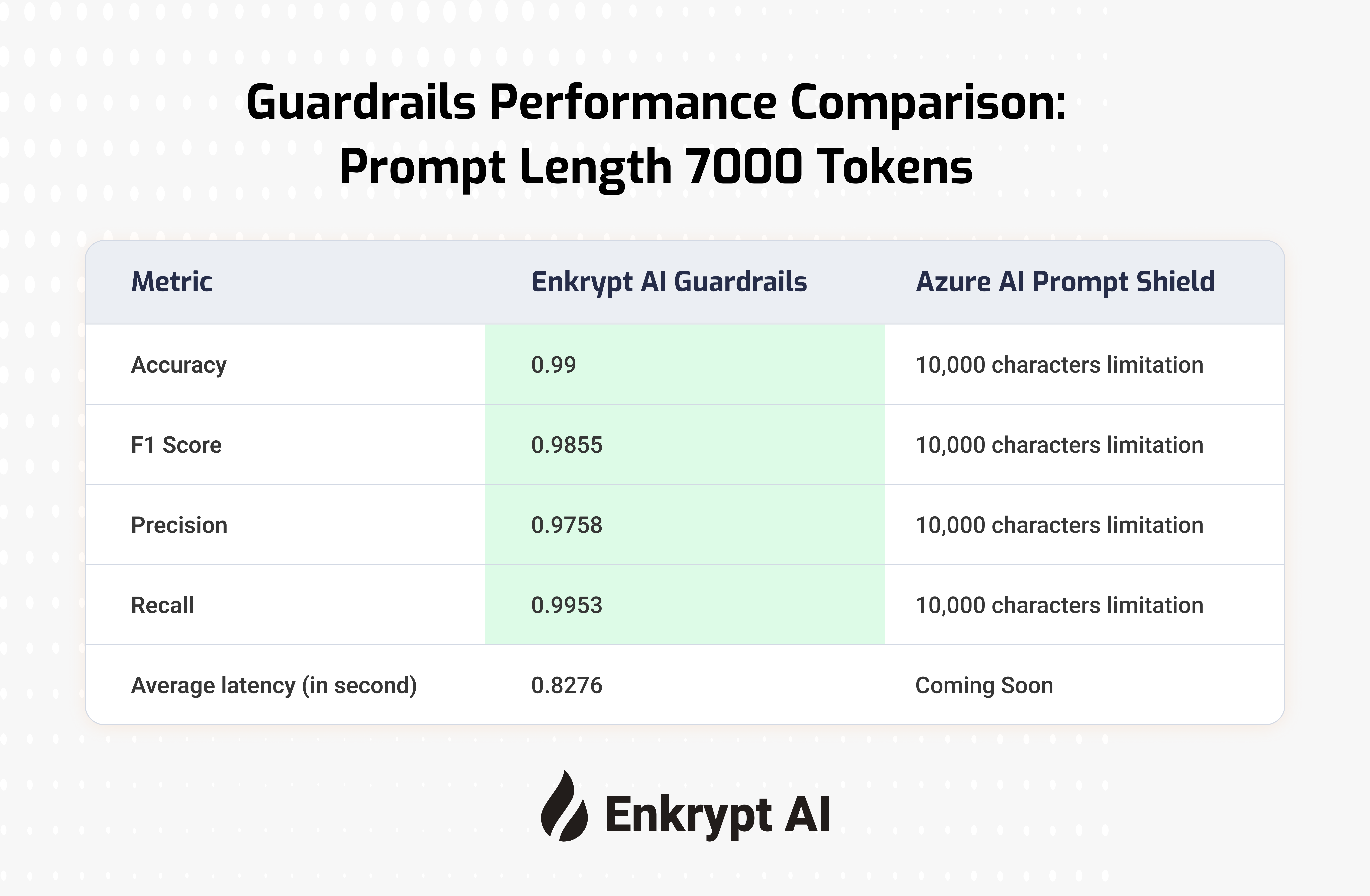
Next, we show the features and different guardrails available from these providers. This comprehensive testing helps organizations understand how guardrails will perform across diverse use cases. See Figure 6 below.
.png)
Comparative Insights
See the overall comparison of the 4 AI Guardrail vendors below.
- Enkrypt AI Guardrails
Strengths:- High Performance: Consistently high scores across accuracy, precision, recall, and F1 metrics, showcasing robust risk mitigation capabilities.
- Feature Richness: Advanced tools like a policy adherence detector, debias detector, hallucination detector, and request relevance checker offer unparalleled comprehensiveness.
- Low Latency: Minimal processing delays make it ideal for real-time, user-facing applications.
- Compliance Focus: Strong adherence to both regulatory and ethical standards, ensuring AI outputs are fair and compliant.
- Best For: Organizations prioritizing comprehensive protection and scalability.
- IBM Granite Guardrails
Strengths:- Accuracy and Recall: Excellent at identifying risks, especially for complex prompts.
- Stable Core Features: Solid moderation and compliance capabilities for general applications.
- Azure AI Prompt Shield
Strengths:- Improved Moderation: Notable advancements in filtering inappropriate content.
- Integration Capabilities: Seamless compatibility with the Microsoft Azure ecosystem.
- Best For: Organizations already using Azure services and dealing with small to medium-sized prompts.
- AWS Bedrock Guardrails
Strengths:- Accuracy and Precision: Strong F1 scores and minimal false positives.
- Broad Use Cases Offers reliable performance for a variety of applications.
Performance Metrics Overview
Refer to table 1for the performance stats for each Guardrail vendor.

Key Takeaways
- Enkrypt AI Guardrails: Offer the most comprehensive protection and scalability, ideal for organizations requiring advanced security and compliance features.
- IBM Granite: Delivers strong performance in recall and stability but lags slightly in precision and latency.
- Azure AI Prompt Shield: Best suited for small to moderate applications but faces challenges with larger workloads.
- AWS Bedrock: A strong contender for accuracy and precision but needs improvement in recall and latency.
Conclusion: Choosing the Right Guardrail Provider
Selecting a guardrail provider depends on the unique needs and risk profile of your organization:
- For comprehensive protection and real-time applications, Enkrypt AI is the clear leader.
- If recall is a primary concern, IBM Granite provides reliable solutions.
- Organizations within the Azure ecosystem may benefit from the integration-friendly Azure AI Prompt Shield.
In the ever-evolving landscape of AI safety, organizations must evaluate their guardrail options thoughtfully, ensuring their LLM deployments are secure, compliant, and effective. Staying updated on technological advancements and tailoring solutions to specific needs will empower organizations to harness the full potential of AI responsibly.